02 April 2025
5 Myths About Forecast Accuracy
Demand forecasting is a crucial component of supply chain management, helping businesses plan inventory, production, and distribution effectively. Many companies strive for higher forecast accuracy, believing it to be the key to improving service levels and reducing costs. However, misconceptions about what forecast accuracy truly means and how it impacts business performance often lead to misaligned strategies and unrealistic expectations.
In this article, we debunk five common myths about forecast accuracy and provide insights on how businesses can develop a more effective, data-driven approach to forecasting.
Myth #1: Higher Forecast Accuracy Always Leads to Better Business Performance
Reality: Forecast accuracy alone does not guarantee better supply chain performance. Agility and responsiveness are equally, if not more, important.
Many organizations assume that improving forecast accuracy will automatically translate into better financial and operational results. While accurate forecasts help align supply with demand, real-world supply chains face unpredictable disruptions supplier delays, sudden demand spikes, and external shocks like geopolitical events or weather-related disruptions.
A retailer with high forecast accuracy might still experience stockouts if their supply chain lacks the flexibility to respond quickly to demand fluctuations. Conversely, a company with only medium forecast accuracy but a highly responsive replenishment system can outperform competitors by swiftly adapting to changing demand.
Instead of solely focusing on forecast accuracy, businesses should invest in supply chain agility—improving lead times, building buffer capacity, and leveraging demand sensing technologies. For example, a company with 95% forecast accuracy but inadequate safety stock might still face frequent stockouts if demand variability is high. Consider a scenario where the expected demand is 100 units, and the safety stock is set at only 5 units. If an unexpected surge pushes demand to 110 units, the company experiences a stockout despite its highly accurate forecast.
Organizations should focus on holistic supply chain resilience & agility than just improving forecast precision.
Myth #2: More Data Automatically Improves Forecast Accuracy
Reality: More data does not necessarily lead to better forecasts; data quality, relevance, and the right analytical models matter more.
With the rise of big data, many companies believe that simply collecting more data (social media trends, weather patterns & customer browsing behaviour) will enhance forecast accuracy. However, more data can introduce noise, biases, and computational challenges if not properly processed and interpreted.
A manufacturer relying solely on historical sales data may miss crucial shifts in market demand due to sudden economic downturns or regulatory changes. Without incorporating external macroeconomic indicators or qualitative business insights, even the most data-rich forecast may fail to provide meaningful accuracy.
Organizations should focus on the right data—clean, structured, and relevant to the forecasting model. Advanced techniques like machine learning can enhance forecasting, but only when combined with domain expertise and business intelligence.
Myth #3: A Single Forecasting Model is Enough for All Situations
Reality: Different products, markets, and supply chain conditions require different forecasting approaches.
There is no one-size-fits-all forecasting model. Factors such as product life cycle stage, demand variability, and supply constraints all influence which forecasting method is most suitable.
- A stable-demand product (e.g., household essentials) may benefit from traditional time-series models like ARIMA or exponential smoothing.
- A new product launch with no historical data requires probabilistic forecasting, analog methods, or judgmental forecasting.
- Highly seasonal products (e.g., winter clothing) require seasonal decomposition models combined with machine learning techniques.
Companies should adopt a hybrid forecasting approach, leveraging multiple models and continuously evaluating their effectiveness based on business needs. Scenario planning can also help mitigate the risks associated with forecast errors.
Myth #4: Forecast Accuracy is Only About Reducing Errors
Reality: The objective is not just to minimize forecast errors but to optimize decision-making under uncertainty.
Many businesses obsess over reducing forecast error percentages without considering the practical trade-offs in supply chain decision-making. A small forecast error can still lead to significant disruptions if the supply chain lacks resilience, while a slightly higher error rate may be acceptable if inventory strategies compensate for the uncertainty.
A company that has 75% accuracy but has flexible vendor contracts and dynamic inventory positioning may perform better than a company that has 85% accuracy and suffers frequent stockouts.
Forecast accuracy should be measured in conjunction with business impact—evaluating metrics such as service levels, inventory holding costs, and customer satisfaction. Companies should aim for decision-centric forecasting rather than just error reduction.
Myth #5: Technology Alone Can Solve Forecasting Challenges
Reality: Advanced AI and forecasting tools enhance accuracy, but human expertise and business judgment remain critical.
With the advent of AI-powered forecasting solutions, many companies believe that technology alone can eliminate forecasting errors. While AI and machine learning can significantly improve accuracy by identifying complex patterns, they still require expert oversight, validation, and integration with business processes.
An AI forecasting model may predict a demand surge based on historical trends, but without business input, it may fail to account for unexpected events like a competitor's aggressive promotional campaign or sudden regulatory changes.
Successful forecasting requires a blend of technology, human expertise, and process discipline. Businesses should invest in training teams to interpret and refine AI-generated forecasts while incorporating real-world business intelligence.
Conclusions: Moving Beyond Forecast Accuracy Myths
Forecasting is a vital tool in supply chain management, but misconceptions about accuracy can lead to ineffective strategies. To build a more resilient and responsive supply chain, businesses should:
- Focus on agility and execution, not just accuracy percentages.
- Prioritize relevant, high-quality data over sheer data volume.
- Use multiple forecasting models tailored to different scenarios.
- Evaluate forecast effectiveness based on business impact, not just error rates.
- Integrate AI with expert-driven decision-making for the best results.
By moving beyond these myths, businesses can adopt a practical, strategic approach to forecasting—one that enhances decision-making, improves service levels, and optimizes supply chain performance.
How We Can Help
At BCI Global we specialize in advanced supply chain analytics, demand forecasting, and inventory optimization. Our team helps businesses develop customized forecasting and inventory optimization strategies that drive real results. Contact us today to learn how we can enhance your forecasting capabilities and improve supply chain resilience.
More information: Jayakhanthan.sakthivel@bciglobal.com
Our related contact is based in Singapore.
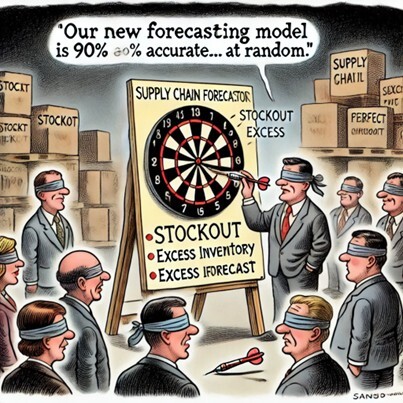